Books
The Interviewer For Your Dream Job Might Soon Be A Robot
As Noreena Hertz reveals in this excerpt from her new book, The Lonely Century, the process is just as alienating as it sounds.
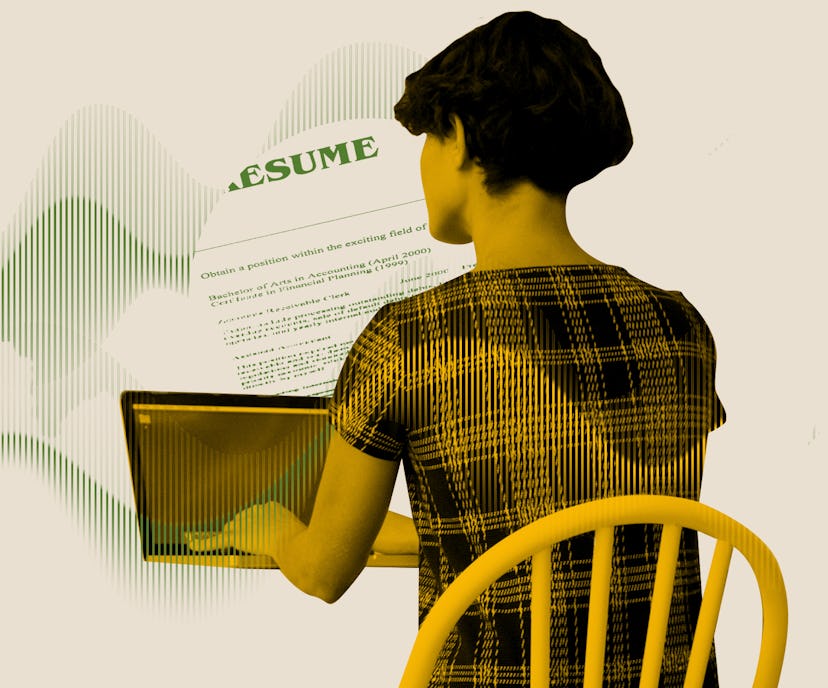
I'm applying for a job. But the application process is one I've never experienced before.
I'm not being interviewed by a person. Instead, I'm sitting at home, staring at my laptop. My answers are being video-recorded. And whether I succeed in getting this job will be determined not by a human being, but by a machine.
This may sound like an episode of Charlie Brooker's Black Mirror, yet within just a few years it is expected that these kind of virtual interviews will be the norm. Algorithmic "pre-hire assessments," as they are called, are already a multi-billion-dollar business and are likely to become a fixture of corporate hiring decisions. HireVue — the company conducting my interview — is one of the leaders in the field. Headquartered along the banks of the Jordan River in Utah, its clients include seven hundred blue-chip companies, from Hilton Hotels to J.P. Morgan to Unilever. I am just one of over 10 million potential employees HireVue's algorithms have already assessed on the basis of similar video interviews.
This is how their artificial intelligence technology worked at the time I took my interview: deploying the next frontier of AI — "emotional AI" — it "reads" job candidates by analyzing their lexicon, tone, cadence, and facial expressions, taking into account as many as twenty-five thousand separate data points. The results were then compared to those of an "ideal" candidate for the role.
The fact that I had to keep my on-screen torso firmly within a dotted-line silhouette throughout the interview meant that not only did I feel like a murder victim in a crime scene, but I couldn't be my authentic self.
In practice what this means is that each breath I took, each pause, the height I raise my eyebrows, how tightly I clenched my jaw, how broad my smile, my choice of words, how loudly I spoke, my posture, how many times I said um or er, my accent, even my preposition usage were all being recorded and fed into a black box algorithm to determine whether or not I was a suitable hire for Vodafone's graduate trainee program. Or rather, not me, but "Irina Wertz," my undercover pseudonym.
Algorithmic pre-hire assessments are undeniably a cost-effective solution to hiring needs at scale. Given that big corporations receive well over one hundred thousand applicants each year, the use of this technology is likely already saving thousands of man-hours. Moreover, HireVue claims that retention rates and even job performance among employees selected by their system are significantly higher than average. This may be so, yet my experience of the process felt more than a little alienating.
As I replied to the questions I was watching myself do so in the corner of the screen, the experience felt especially performative, with me cast in the disquieting role of both actor and audience.
The fact that I had to keep my on-screen torso firmly within a dotted-line silhouette throughout the interview meant that not only did I feel like a murder victim in a crime scene, but I couldn't be my authentic self. Some degree of inauthenticity is of course inevitable in all job interviews, given that one tries to present a crafted, best-possible version of oneself, but this was different. I am an expressive person — I move when I speak, I gesticulate. Stuck in my silhouette, I couldn't even do that. And because as I replied to the questions I was watching myself do so in the corner of the screen, the experience felt especially performative, with me cast in the disquieting role of both actor and audience.
At the top right of the screen was a countdown clock which added to the stressful nature of the experience. I was allocated three minutes to answer each question, but flying blind without all the usual cues one gets from a human interviewer — facial expressions, head movements, gestures, smiles, frowns — I wasn't sure whether I was going on too long, or whether I was expected to use up all of the time. And not only did I have no one to ask, but with no smiles, no eyes darting down to my CV, no body language to parse, I couldn't tell if my "interviewer" had heard enough of a particular answer, liked what I was saying, understood my jokes, empathized with my stories, or maybe had just decided that I was not the kind of candidate they were looking for.
Stripped of my full, complex humanity, I had to impress a machine whose black-box algorithmic workings I could never know. Which of my "data points" was it focusing on and which was it weighting the most heavily?
So as the interview proceeded, I felt increasingly adrift, unable to figure out whether to keep going, slow down, shift gears, change tack, alter my style, smile more, smile less. Presumably the ideal candidate for a graduate traineeship in human resources at Vodafone smiles, but how many times and for how long?
To be clear, it wasn't that I was interacting with a machine per se that made me feel so alienated. Rather, it was the power imbalance between woman and machine that was so troubling. Stripped of my full, complex humanity, I had to impress a machine whose black-box algorithmic workings I could never know. Which of my "data points" was it focusing on and which was it weighting the most heavily? My voice, my intonation, my body language, or the content of what I was saying? What formula was it using to assess me? And was it fair?
Its algorithm will have been trained on video footage of past or existing "successful hires," which means that any historic biases (conscious or unconscious) in hiring would likely be replicated.
We don't normally think about loneliness in the context of how an interaction with a machine makes us feel. When I talked about the isolation of a contactless existence, my emphasis was on the lack of face-to-face human contact and its impact. But if loneliness can also be caused by a feeling of being unfairly treated and disempowered by the state and by politicians, so too can it stem from being treated as such by Big Business and the new technologies it deploys.
For when an employer puts our professional futures in the hands of an algorithm, it is hard to believe that we'll be treated fairly or have meaningful recourse. In part this is because it is highly contestable as to whether future performance can actually be determined by characteristics such as facial expressions and tone of voice. Indeed, in November 2019 the Electronic Privacy Information Center — a renowned U.S. public interest research organization — filed a formal complaint against HireVue with the U.S. Federal Trade Commission, citing HireVue's "use of secret, unproven algorithms to assess the 'cognitive ability,' 'psychological traits,' 'emotional intelligence,' and 'social aptitudes' of job candidates." Interestingly since early 2020 the company has stopped using facial analysis, concluding that “visual analysis has less correlation to job performance than other elements of of algorithmic assessment”. Other companies however continue to do so.
The artificial-intelligence CV-sorter had effectively taught itself that applications that included the names of all-women colleges or even the word women's ("captain of the women's chess team," for example) were unqualified.
There's also the question of bias. For although HireVue claimed at the time that its methodology gets rid of human bias, this is unlikely to be so. This is because algorithms are trained on video footage of past or existing "successful hires," which means that any historic biases (conscious or unconscious) in hiring would likely be replicated.
This is in fact precisely what happened at Amazon in 2018, when it was revealed that the company's artificial-intelligence CV-sorter was routinely rejecting women's CVs, despite never being "told" the applicants' gender. Why? It had effectively taught itself that applications that included the names of all-women colleges or even the word women's ("captain of the women's chess team," for example) were unqualified. This was because it had been trained to deduce whether applicants were "qualified" or "unqualified" on the basis of ten years of hiring data in an industry where men make up the vast majority of applicants and hires. Needless to say, there were very few captains of women's chess teams in that group.
Adjusting an algorithm to address biases as obvious as gender is relatively straightforward; indeed, Amazon's engineers were easily able to edit the model to stop using terms like women's as a reason for disqualification. But the challenge with machine learning is that even if the most obvious sources of bias are accounted for (and doubtless are, in many such systems), what about less obvious, neutral-seeming data points that one might not even consider could be biased?
Excerpted from The Lonely Century: How To Restore Human Connection In A World That's Pulling Apart, published by Currency, an imprint of Penguin Random House.
Ed's Note: This extract has been updated to reflect that HireVue no longer uses visual analysis in its hiring software.